Latest posts for tag python
Gtk4 has interesting ways of splitting models and views. One that I didn't find very well documented, especially for Python bindings, is a set of radio buttons backed by a common model.
The idea is to define an action that takes a string as a state. Each radio button is assigned a string matching one of the possible states, and when the state of the backend action is changed, the radio buttons are automatically updated.
All the examples below use a string for a value type, but anything can be used
that fits into a GLib.Variant
.
The model
This defines the action. Note that enables all the usual declarative ways of a status change:
mode = Gio.SimpleAction.new_stateful(
name="mode-selection",
parameter_type=GLib.VariantType("s"),
state=GLib.Variant.new_string(""))
gtk_app.add_action(self.mode)
The view
def add_radio(model: Gio.SimpleAction, id: str, label: str):
button = Gtk.CheckButton(label=label)
# Tell this button to activate when the model has the given value
button.set_action_target_value(GLib.Variant.new_string(id))
# Build the name under which the action is registesred, plus the state
# value controlled by this button: clicking the button will set this state
detailed_name = Gio.Action.print_detailed_name(
"app." + model.get_name(),
GLib.Variant.new_string(id))
button.set_detailed_action_name(detailed_name)
# If the model has no current value set, this sets the first radio button
# as selected
if not model.get_state().get_string():
model.set_state(GLib.Variant.new_string(id))
Accessing the model
To read the currently selected value:
current = model.get_state().get_string()
To set the currently selected value:
model.set_state(GLib.Variant.new_string(id))
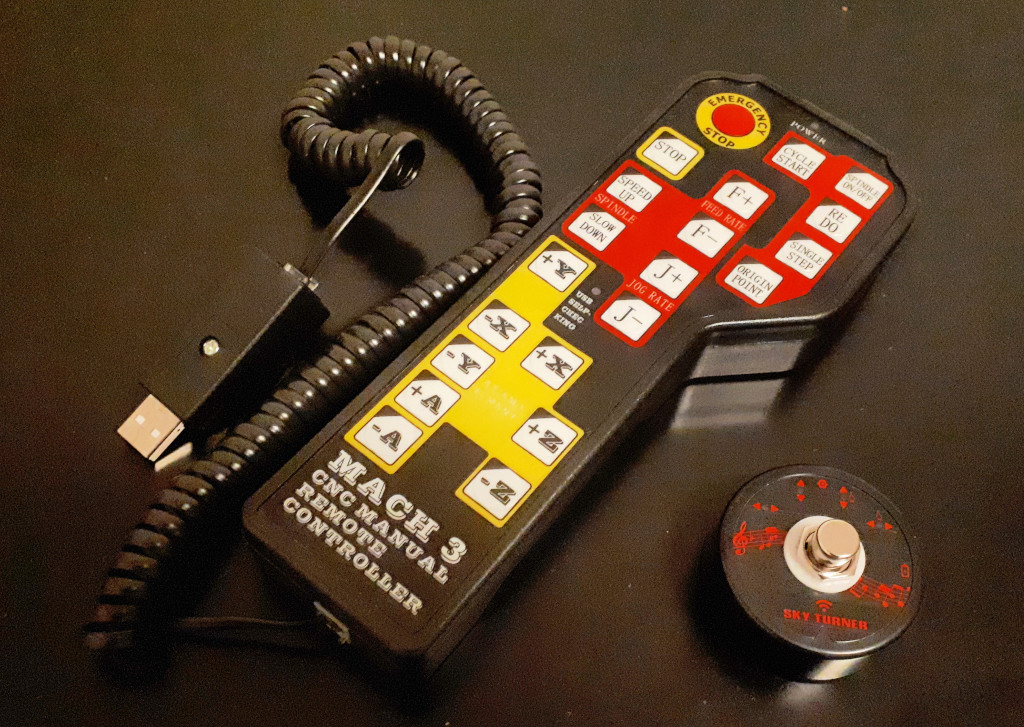
I acquired some unusual input devices to experiment with, like a CNC control panel and a bluetooth pedal page turner.
These identify and behave like a keyboard, sending nice and simple keystrokes, and can be accessed with no drivers or other special software. However, their keystrokes appear together with keystrokes from normal keyboards, which is the expected default when plugging in a keyboard, but not what I want in this case.
I'd also like them to be readable via evdev and accessible by my own user.
Here's the udev rule I cooked up to handle this use case:
# Handle the CNC control panel
SUBSYSTEM=="input", ENV{ID_VENDOR}=="04d9", ENV{ID_MODEL}=="1203", \
OWNER="enrico", ENV{ID_INPUT}=""
# Handle the Bluetooth page turner
SUBSYSTEM=="input", ENV{ID_BUS}=="bluetooth", ENV{LIBINPUT_DEVICE_GROUP}=="*/…mac…", ENV{ID_INPUT_KEYBOARD}="1" \
OWNER="enrico", ENV{ID_INPUT}="", SYMLINK+="input/by-id/bluetooth-…mac…-kbd"
SUBSYSTEM=="input", ENV{ID_BUS}=="bluetooth", ENV{LIBINPUT_DEVICE_GROUP}=="*/…mac…", ENV{ID_INPUT_TABLET}="1" \
OWNER="enrico", ENV{ID_INPUT}="", SYMLINK+="input/by-id/bluetooth-…mac…-tablet"
The bluetooth device didn't have standard rules to create /dev/input/by-id/
symlinks so I added them. In my own code, I watch /dev/input/by-id
with
inotify to handle when devices appear or disappear.
I used udevadm info /dev/input/event…
to see what I could use to identify the
device.
The Static device configuration via udev page of libinput's documentation has documentation on the various elements specific to the input subsystem
Grepping rule files in /usr/lib/udev/rules.d
was useful to see syntax
examples.
udevadm test /dev/input/event…
was invaluable for syntax checking and testing
my rule file while working on it.
Finally, this is an extract of a quick prototype Python code to read keys from the CNC control panel:
import libevdev
KEY_MAP = {
libevdev.EV_KEY.KEY_GRAVE: "EMERGENCY",
# InputEvent(EV_KEY, KEY_LEFTALT, 1)
libevdev.EV_KEY.KEY_R: "CYCLE START",
libevdev.EV_KEY.KEY_F5: "SPINDLE ON/OFF",
# InputEvent(EV_KEY, KEY_RIGHTCTRL, 1)
libevdev.EV_KEY.KEY_W: "REDO",
# InputEvent(EV_KEY, KEY_LEFTALT, 1)
libevdev.EV_KEY.KEY_N: "SINGLE STEP",
# InputEvent(EV_KEY, KEY_LEFTCTRL, 1)
libevdev.EV_KEY.KEY_O: "ORIGIN POINT",
libevdev.EV_KEY.KEY_ESC: "STOP",
libevdev.EV_KEY.KEY_KPPLUS: "SPEED UP",
libevdev.EV_KEY.KEY_KPMINUS: "SLOW DOWN",
libevdev.EV_KEY.KEY_F11: "F+",
libevdev.EV_KEY.KEY_F10: "F-",
libevdev.EV_KEY.KEY_RIGHTBRACE: "J+",
libevdev.EV_KEY.KEY_LEFTBRACE: "J-",
libevdev.EV_KEY.KEY_UP: "+Y",
libevdev.EV_KEY.KEY_DOWN: "-Y",
libevdev.EV_KEY.KEY_LEFT: "-X",
libevdev.EV_KEY.KEY_RIGHT: "+X",
libevdev.EV_KEY.KEY_KP7: "+A",
libevdev.EV_KEY.KEY_Q: "-A",
libevdev.EV_KEY.KEY_PAGEDOWN: "-Z",
libevdev.EV_KEY.KEY_PAGEUP: "+Z",
}
class KeyReader:
def __init__(self, path: str):
self.path = path
self.fd: IO[bytes] | None = None
self.device: libevdev.Device | None = None
def __enter__(self):
self.fd = open(self.path, "rb")
self.device = libevdev.Device(self.fd)
return self
def __exit__(self, exc_type, exc, tb):
self.device = None
self.fd.close()
self.fd = None
def events(self) -> Iterator[dict[str, Any]]:
for e in self.device.events():
if e.type == libevdev.EV_KEY:
if (val := KEY_MAP.get(e.code)):
yield {
"name": val,
"value": e.value,
"sec": e.sec,
"usec": e.usec,
}
Edited: added rules to handle the Bluetooth page turner
- str.endswith() can take a tuple of possible endings instead of a single string
About JACK and Debian
- There are 3 JACK implementations: jackd1, jackd2, pipewire-jack.
- jackd1 is mostly superseded in favour of jackd2, and as far as I understand, can be ignored
- pipewire-jack integrates well with pipewire and the rest of the Linux audio world
- jackd2 is the native JACK server. When started it handles the sound card directly, and will steal it from pipewire. Non-JACK audio applications will likely cease to see the sound card until JACK is stopped and wireplumber is restarted. Pipewire should be able to keep working as a JACK client but I haven't gone down that route yet
- pipewire-jack mostly works. At some point I experienced glitches in complex JACK apps like giada or ardour that went away after switching to jackd2. I have not investigated further into the glitches
- So: try things with pw-jack. If you see odd glitches, try without pw-jack to use the native jackd2. Keep in mind, if you do so, that you will lose standard pipewire until you stop jackd2 and restart wireplumber.
I have Python code for reading a heart rate monitor.
I have Python code to generate MIDI events.
Could I resist putting them together? Clearly not.
Here's Jack Of Hearts, a JACK MIDI drum loop generator that uses the heart rate for BPM, and an improvised way to compute heart rate increase/decrease to add variations in the drum pattern.
It's very simple minded and silly. To me it was a fun way of putting unrelated things together, and Python worked very well for it.
I had a go at trying to figure out how to generate arbitrary MIDI events and send them out over a JACK MIDI channel.
Setting up JACK and Pipewire
Pipewire has a JACK interface, which in theory means one could use JACK clients out of the box without extra setup.
In practice, one need to tell JACK clients which set of libraries to use to communicate to servers, and Pipewire's JACK server is not the default choice.
To tell JACK clients to use Pipewire's server, you can either:
- on a client-by-client basis, wrap the commands with pw-jack
- to change the system default:
cp /usr/share/doc/pipewire/examples/ld.so.conf.d/pipewire-jack-*.conf /etc/ld.so.conf.d/
and runldconfig
(see the Debian wiki for details)
Programming with JACK
Python has a JACK client library that worked flawlessly for me so far.
Everything with JACK is designed around minimizing latency. Everything happens around a callback that gets called form a separate thread, and which gets a buffer to fill with events.
All the heavy processing needs to happen outside the callback, and the callback is only there to do the minimal amount of work needed to shovel the data your application produced into JACK channels.
Generating MIDI messages
The Mido library can be used to parse and create MIDI messages and it also worked flawlessly for me so far.
One needs to study a bit what kind of MIDI message one needs to generate (like "note on", "note off", "program change") and what arguments they get.
It also helps to read about the General MIDI standard which defines mappings between well-known instruments and channels and instrument numbers in MIDI messages.
A timed message queue
To keep a queue of events that happen over time, I implemented a Delta List that indexes events by their future frame number.
I called the humble container for my audio experiments pyeep and here's my delta list implementation.
A JACK player
The simple JACK MIDI player backend is also in pyeep.
It needs to protect the delta list with a mutex since we are working across thread boundaries, but it tries to do as little work under lock as possible, to minimize the risk of locking the realtime thread for too long.
The play
method converts delays in seconds to frame counts, and the
on_process
callback moves events from the queue to the jack output.
Here's an example script that plays a simple drum pattern:
#!/usr/bin/python3
# Example JACK midi event generator
#
# Play a drum pattern over JACK
import time
from pyeep.jackmidi import MidiPlayer
# See:
# https://soundprogramming.net/file-formats/general-midi-instrument-list/
# https://www.pgmusic.com/tutorial_gm.htm
DRUM_CHANNEL = 9
with MidiPlayer("pyeep drums") as player:
beat: int = 0
while True:
player.play("note_on", velocity=64, note=35, channel=DRUM_CHANNEL)
player.play("note_off", note=38, channel=DRUM_CHANNEL, delay_sec=0.5)
if beat == 0:
player.play("note_on", velocity=100, note=38, channel=DRUM_CHANNEL)
player.play("note_off", note=36, channel=DRUM_CHANNEL, delay_sec=0.3)
if beat + 1 == 2:
player.play("note_on", velocity=100, note=42, channel=DRUM_CHANNEL)
player.play("note_off", note=42, channel=DRUM_CHANNEL, delay_sec=0.3)
beat = (beat + 1) % 4
time.sleep(0.3)
Running the example
I ran the jack_drums
script, and of course not much happened.
First I needed a MIDI synthesizer. I installed fluidsynth, and ran it on the command line with no arguments. it registered with JACK, ready to do its thing.
Then I connected things together. I used qjackctl, opened the graph view, and connected the MIDI output of "pyeep drums" to the "FLUID Synth input port".
fluidsynth's output was already automatically connected to the audio card and I started hearing the drums playing! 🥁️🎉️
I bought myself a cheap wearable Bluetooth LE heart rate monitor in order to play with it, and this is a simple Python script to monitor it and plot data.
Bluetooth LE
I was surprised that these things seem decently interoperable.
You can use hcitool
to scan for devices:
hcitool lescan
You can then use gatttool
to connect to device and poke at them interactively
from a command line.
Bluetooth LE from Python
There is a nice library called Bleak which is also packaged in Debian. It's modern Python with asyncio and works beautifully!
Heart rate monitors
Things I learnt:
- The UUID for the heart rate interface starts with
00002a37
. - The UUID for checking battery status starts with
00002a19
. - A longer list of UUIDs is here.
- The layout of heart rate data packets and some Python code to parse them
- What are RR values
How about a proper fitness tracker?
I found OpenTracks, also on F-Droid, which seems nice
Why script it from a desktop computer?
The question is: why not?
A fitness tracker on a phone is useful, but there are lots of silly things one can do from one's computer that one can't do from a phone. A heart rate monitor is, after all, one more input device, and there are never enough input devices!
There are so many extremely important use cases that seem entirely unexplored:
- Log your heart rate with your git commits!
- Add your heart rate as a header in your emails!
- Correlate heart rate information with your work activity tracker to find out what tasks stress you the most!
- Sync ping intervals with your own heartbeat, so you get faster replies when you're more anxious!
- Configure workrave to block your keyboard if you get too excited, to improve the quality of your mailing list contributions!
- You can monitor the monitor script of the heart rate monitor that monitors you! Forget buffalo, be your monitor monitor monitor monitor monitor monitor monitor monitor...
In theory I wanted to announce the release of
staticsite 2.0, but then I found
bugs that prevented me from writing this post, so I'm also releasing
2.1 2.2 2.3 :grin:
staticsite is the static site generator that I ended up writing after giving other generators a try.
I did a big round of cleanup of the code, which among other things allowed me to implement incremental builds.
It turned out that staticsite is fast enough that incremental builds are not really needed, however, a bug in caching rendered markdown made me forget about that. Now I fixed that bug, too, and I can choose between running staticsite fast, and ridiculously fast.
My favourite bit of this work is the internal cleanup: I found a way to simplify the core design massively, and now the core and plugin system is simple enough that I can explain it, and I'll probably write a blog post or two about it in the next days.
On top of that, staticsite is basically clean with mypy running in strict mode! Getting there was a great ride which prompted a lot of thinking about designing code properly, as mypy is pretty good at flagging clumsy hacks.
If you want to give it a try, check out the small tutorial A new blog in under one minute.
argparse subcommands are great, but they have a quirk in which options are only available right after the subcommand that define them.
So, if you for example add the --verbose / -v
argument to your main parser,
and you have subcommands, you need to give the -v
option before the
subcommand name. For example, given this script:
#!/usr/bin/python3
import argparse
parser = argparse.ArgumentParser(description="test")
parser.add_argument("-v", "--verbose", action="store_true")
subparsers = parser.add_subparsers(dest="handler", required=True)
subparsers.add_parser("test")
args = parser.parse_args()
print(args.verbose)
You get this behaviour:
$ ./mycmd test
False
$ ./mycmd -v test
True
$ ./mycmd test -v
usage: mycmd [-h] [-v] {test} ...
mycmd: error: unrecognized arguments: -v
This sometimes makes sense, and many other times it's really annoying, since the user has to remember at which level an option was defined.
Last night some pieces clicked in my head, and I created a not-too-dirty
ArgumentParser subclass
that adds a shared
option to arguments, that propagates them to subparsers:
#!/usr/bin/python3
from hacks import argparse
parser = argparse.ArgumentParser(description="test")
parser.add_argument("-v", "--verbose", action="store_true", shared=True)
subparsers = parser.add_subparsers(dest="handler", required=True)
subparsers.add_parser("test")
args = parser.parse_args()
print(args.verbose)
And finally, -v
can be given at all levels:
$ ./mycmd test
False
$ ./mycmd -v test
True
$ ./mycmd test -v
True
It even works recursively, forwarding arguments to sub-subparsers, but at the
moment it can only do it for store_true
and store
kind of arguments.
This is a common logging pattern in Python, to have loggers related to module names:
import logging
log = logging.getLogger(__name__)
class Bill:
def load_bill(self, filename: str):
log.info("%s: loading file", filename)
I often however find myself wanting to have loggers related to something
context-dependent, like the kind of file that is being processed. For example,
I'd like to log loading of bill loading when done by the expenses
module, and
not when done by the printing
module.
I came up with a little hack that keeps the same API as before, and allows to propagate a context dependent logger to the code called:
# Call this file log.py
from __future__ import annotations
import contextlib
import contextvars
import logging
_log: contextvars.ContextVar[logging.Logger] = contextvars.ContextVar('log', default=logging.getLogger())
@contextlib.contextmanager
def logger(name: str):
"""
Set a default logger for the duration of this context manager
"""
old = _log.set(logging.getLogger(name))
try:
yield
finally:
_log.reset(old)
def debug(*args, **kw):
_log.get().debug(*args, **kw)
def info(*args, **kw):
_log.get().info(*args, **kw)
def warning(*args, **kw):
_log.get().warning(*args, **kw)
def error(*args, **kw):
_log.get().error(*args, **kw)
And now I can do this:
from . import log
# …
with log.logger("expenses"):
bill = load_bill(filename)
# This code did not change!
class Bill:
def load_bill(self, filename: str):
log.info("%s: loading file", filename)
Suppose you have a tool that archives images, or scientific data, and it has a test suite. It would be good to collect sample files for the test suite, but they are often so big one can't really bloat the repository with them.
But does the test suite need everything that is in those files? Not necesarily. For example, if one's testing code that reads EXIF metadata, one doesn't care about what is in the image.
That technique works extemely well. I can take GRIB files that are several megabytes in size, zero out their data payload, and get nice 1Kb samples for the test suite.
I've started to collect and organise the little hacks I use for this into a tool I called mktestsample:
$ mktestsample -v samples1/*
2021-11-23 20:16:32 INFO common samples1/cosmo_2d+0.grib: size went from 335168b to 120b
2021-11-23 20:16:32 INFO common samples1/grib2_ifs.arkimet: size went from 4993448b to 39393b
2021-11-23 20:16:32 INFO common samples1/polenta.jpg: size went from 3191475b to 94517b
2021-11-23 20:16:32 INFO common samples1/test-ifs.grib: size went from 1986469b to 4860b
Those are massive savings, but I'm not satisfied about those almost 94Kb of JPEG:
$ ls -la samples1/polenta.jpg
-rw-r--r-- 1 enrico enrico 94517 Nov 23 20:16 samples1/polenta.jpg
$ gzip samples1/polenta.jpg
$ ls -la samples1/polenta.jpg.gz
-rw-r--r-- 1 enrico enrico 745 Nov 23 20:16 samples1/polenta.jpg.gz
I believe I did all I could: completely blank out image data, set quality to zero, maximize subsampling, and tweak quantization to throw everything away.
Still, the result is a 94Kb file that can be gzipped down to 745 bytes. Is there something I'm missing?
I suppose JPEG is better at storing an image than at storing the lack of an image. I cannot really complain :)
I can still commit compressed samples of large images to a git repository, taking very little data indeed. That's really nice!